Empowering scientific discovery through AI innovation
Empowering scientific discovery through AI innovation
At Seqera, we believe that AI isn’t just a tool - it’s the future of scientific discovery. We're committed to harnessing its transformative power to revolutionize the Life Sciences. As innovation continues to accelerate, AI has evolved from an experimental technology to a key component, reshaping research and healthcare in ways previously unimaginable. We see AI as the catalyst that will empower scientists to unlock scientific breakthroughs that deepen our understanding of diseases and transform human health.
AI will redefine the future of science, paving the way for advancements that contribute directly to human health. We believe AI is integral to genomics and drug discovery, and we have been pioneering this vision since the inception of Nextflow, now over 10 years ago. Nextflow is a powerful parallelization engine that is used by scientists to run high throughput workloads, process data, generate predictions and even train machine learning models. Nextflow is also a language designed to make it easy to write, debug, generate, and execute code in an LLM-first world.
Seqera’s goal is to advance science for everyone through software. We have strengthened our mission through our recent acquisition of tinybio, which brings us closer to empowering scientists with AI to fuel breakthroughs in genomics, drug discovery, and personalized medicines. As AI becomes a cornerstone of the Life Sciences, we must ask ourselves: How does the emergence of these technologies inspire us to evolve our approach to further support the discoveries and innovations of the future? Can we draw insights from how Nextflow has supported the last 10 years of discoveries and innovations in science to the role Seqera will play going forward?
In this blog post, we will explore the brief history of AI in biomedical research, highlight examples of AI in R&D and clinical settings, discuss best practices for AI in scientific research, and identify emerging trends.
Brief history of AI in biomedical research
The application of AI in biomedicine has evolved significantly over the years, beginning with early efforts in machine learning to identify patterns in large biological datasets. In the early 2000s, AI was used to predict protein structures and model complex biological systems, but these models were often limited by computational power and data availability.
Since then, the landscape has changed dramatically, driven largely by advancements in next-generation sequencing (NGS), high-throughput experiments generating larger datasets and the computational techniques to analyze complex biological data. This has led to the emergence of advanced bioinformatics tools and software solutions. Cloud computing has revolutionized data management by providing scalable resources for handling extensive datasets, while technologies like Docker enable the creation of reproducible analytical workflows across diverse computational environments. This evolution has established the data and infrastructure foundations that facilitate the next generation of AI use cases in science.
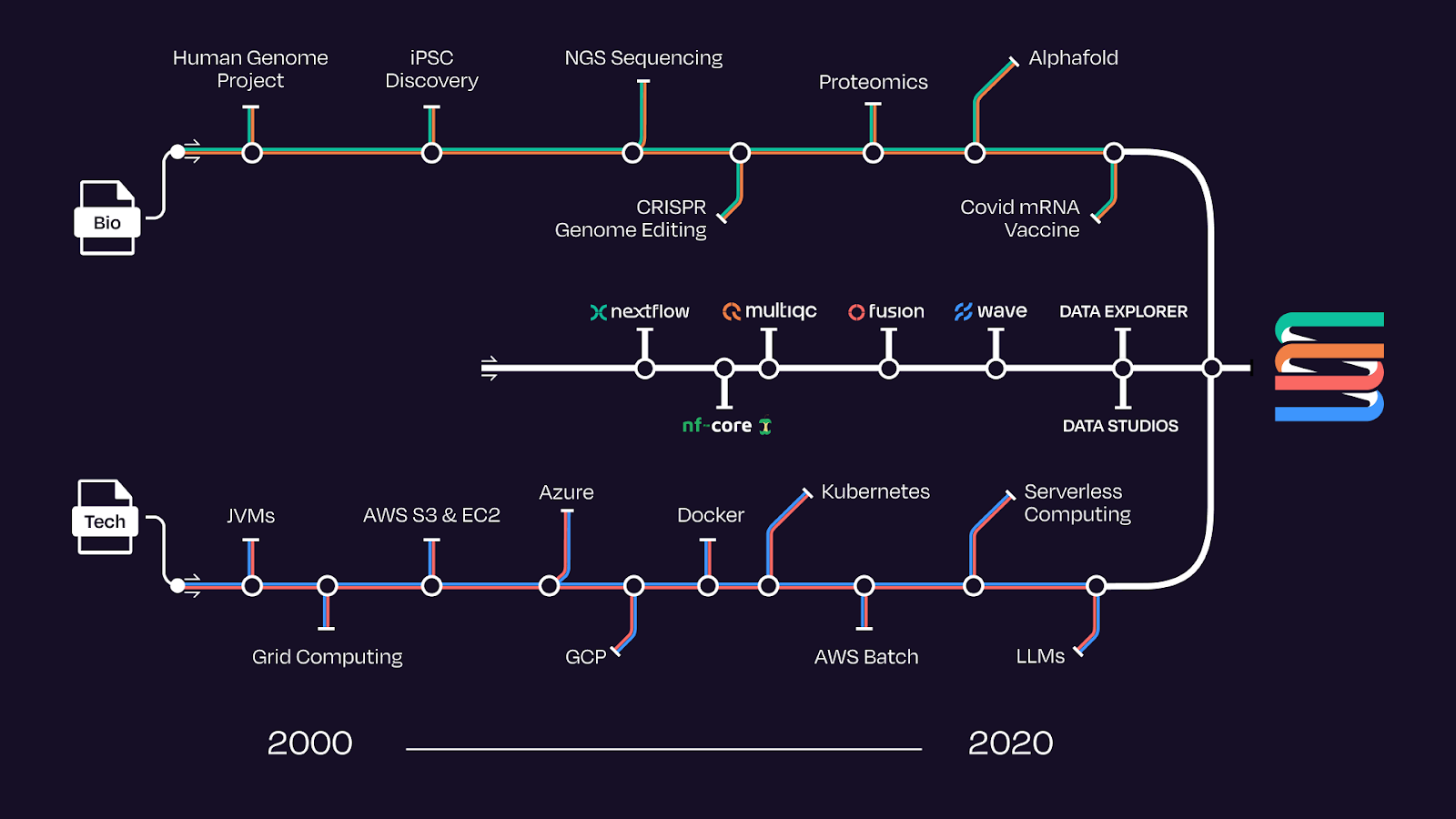
Figure 1. AI the convergence of bio, cloud and software: bringing together advances in science and infrastructure.
Notable advancements, such as AlphaFold2, whose developers won the Nobel Prize in Chemistry in 2024, have revolutionized our ability to predict protein structures, showcasing AI's potential to solve fundamental biological problems. In recent years, AI has expanded its influence across areas like genomics, drug discovery, and personalized medicine. By helping researchers prioritize drug candidates and analyze complex biological pathways with unprecedented speed and accuracy, AI is reshaping the future of biomedicine.
Examples of AI in R&D and the clinic
We are already witnessing the impact of AI in the Life Sciences. The U.S. Food and Drug Administration (FDA) has seen a significant increase in drug and biologic application submissions which use AI/ML components, illustrating the profound impact of these technologies in the drug development lifecycle. Other examples of use in both R&D and the clinic include:
- Knowledge extraction: Through the application of AI and knowledge graphs, Wisecube were able to explore repurposed drugs for Alzheimer's Disease, offering a promising avenue to accelerate scientific breakthroughs in treatments.
- Compound generation: EXS-21546, an AI-designed A2A receptor antagonist developed by Exscientia for immuno-oncological applications, is currently undergoing clinical trials.
- Target prediction - Gritstone Bio have developed an AI-based platform to predict which mutations will generate tumor-specific neoantigens most likely to be presented on the tumor cell surface by human leukocyte antigen (HLA) molecules.
- Patient identification: Using AI, IQVIA were able to increase the precision of patient identification by 15x and healthcare professional linkage by 10x for oncology patients eligible for new first- or second-line therapies.
Many of the breakthroughs in AI-driven biomedicine wouldn’t have been possible without robust computational frameworks to process and analyze massive datasets, like Nextflow. By enabling researchers to scale their data processing workflows efficiently, Nextflow has been pivotal to ensuring reproducibility and flexibility in biomedical research. The same computational rigor that powers large-scale scientific discoveries now supports AI's integration into biomedicine, ensuring that complex models can be run reliably, reproducibly, and at scale — a critical factor in realizing the full potential of AI in the Life Sciences.
AI best practices in scientific research
As we look towards a future increasingly powered by AI we must continue to innovate our products to support more complex workflows that meet the evolving demands of scientific research. We must also prioritize AI best practices to ensure reproducibility and reliability, which is fundamental to scientific integrity. Scientists can seize the opportunity presented by AI’s indeterminism to embrace more rigorous standards, ensuring that AI augments research outcomes without undermining truth and reality. Several best practices are already being adopted by the scientific community, including:
- Open Source code: Promoting transparency through open source across data processing, model training and inference enables everyone to inspect, verify and build upon existing AI models. Examples of open-source projects include DeepChem for drug discovery and OpenBioLink for integrating biological data.
- AI model registries: Creating registries of AI models developed for scientific applications, allowing researchers to document and report any minimal attributes of AI systems. Examples include Hugging Face and TensorFlow Hub, which host a wide range of pre-trained models for tasks like biomedical text mining or image analysis.
- Containerized environments: Containers encapsulate all dependencies needed for a workflow, enabling the standardization and reproducibility of AI-driven research across different systems and platforms. Tools like Docker, Apptainer (formerly Singularity) and Wave facilitate this process, enabling the seamless sharing and deployment of workflows with consistent performance.
- Cloud-based resources: Cloud-based resources can help improve reproducibility and scalability of AI studies by revolutionizing how scientists access and use data in their studies. Cloud-based platforms, including AWS, GCP and Microsoft Azure, provide robust computing power and storage capabilities that enable consistent workflows, large-scale data processing, and enhanced collaboration.
Future directions and emerging trends
From accelerated drug discovery to personalized medicines, AI is set to continue to reshape biomedical R&D. New opportunities are emerging for enhancing the efficiency, accuracy and creativity of scientists, with the aim of ensuring breakthroughs beneficial to humanity and the planet. As AI continues to evolve, several crucial questions will guide future applications:
- Scientific standards for AI: How can we develop and implement AI standards to address challenges related to reproducibility in scientific research?
- Evolving skill sets: How are the skills required for scientific research shifting with the growing adoption of AI, and what new competencies will be needed?
- Expanding accessibility: What obstacles hinder equal access to AI technologies for all scientists, and how can we address them?
- Optimizing AI workflows: How can we enhance existing AI workflows to ensure maximum efficiency in computing infrastructure utilization?
Our commitment to AI best practices and Open Science
At Seqera, we are committed to accelerating scientific discoveries for everyone through software, striving to empower the next generation of researchers by staying at the forefront of innovation and technology. With AI and Open Science, we are continuing to make this a reality. From Nextflow, which is optimized for leveraging LLMs, to our acquisition of tinybio, which democratizes access to essential bioinformatics tools, we want to enable everyone to benefit from advanced data analysis. By integrating AI, we are making these tools smarter, more intuitive, and more accessible than ever.
Want to keep up to date with AI product advancements at Seqera? Register for our upcoming "What's New" product webinar or subscribe to our Newsletter